Fourth International Workshop on
“Robust Subspace Learning and Applications in Computer Vision”
in conjunction with
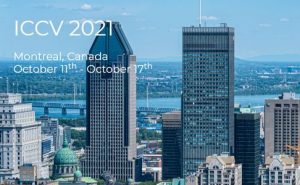
Robust subspace learning/tracking/clustering either based on robust statistics estimation on reconstruction error and on decompositions into low-rank/sparse plus additive matrices/tensors provide suitable frameworks for many computer vision applications like in video coding, key frame extraction, hyper-spectral video processing, dynamic MRI, motion saliency detection, background initialization and background/foreground separation. In this context, the previous workshops RSL-CV hosted at ICCV 2015, ICCV 2017 and ICCV 2019 aimed to propose novel robust subspace clustering/learning/tracking approaches with adaptive and incremental algorithms.
Even if progress have been made since the last decade, there are still main challenges that concern the fundamental design of relaxed models and solvers that have to be with iterations as few as possible, and as efficient as possible. In addition, efforts should concentrated on provable correct algorithms with convergence guarantees as well as robust subspace recovery algorithms. Furthermore, recent advances on low-rank and sparse embedding for dimensionality reduction, robust graph learning and robust deep auto-encoders give promising gap of performance by applying them in computer vision. Finally, even if many efforts have been made to develop methods that perform well visually with reduced computational cost, no algorithm has emerged that is able to simultaneously address all of the key challenges that accompany real world videos taken by static or moving cameras like illumination changes, dynamic backgrounds, bootstrapping that generate corrupted and missing data.
The goals of this workshop are thus three-fold: 1) designing robust subspace methods for computer vision applications; 2) proposing new adaptive and incremental algorithms with convergence guarantees that reach the requirements of real-time applications (motion saliency, video coding and background/foreground separation); and 3) proposing robust algorithms to handle the key challenges in computer vision application.
Papers are solicited to address robust subspace clustering/learning/tracking based on matrix/tensor decomposition, to be applied in computer vision, including but not limited to the followings:
- Robust Subspace Learning: RPCA, RMF, RMC
- Robust Low Rank Factorization
- Approximation/Recovery
- Robust Subspace Tracking
- Robust Subspace Clustering
- Decomposition into low-rank/sparse plus additive
- matrices/tensors
- Bayesian RPCA, Fuzzy RPCA
- Compressive Sensing
- Dictionary Learning
- Structured Sparsity, Dynamic Group Sparsity
- Solvers (ALM, ADM, etc…),
- Closed form solutions
- Efficient SVD algorithms
- Multilevel RPCA
- Incremental RPCA
- Real time implementation on GPU
- Embedded implementation
- Deep Learning
- Robust Deep Auto-Encoder
- Visible spectrum
- IR Imaging
- Hyperspectral imaging
- SAR Imaging
Other resources are available here.
The printable Call for Papers for RSL-CV 2021 can be downloaded here.